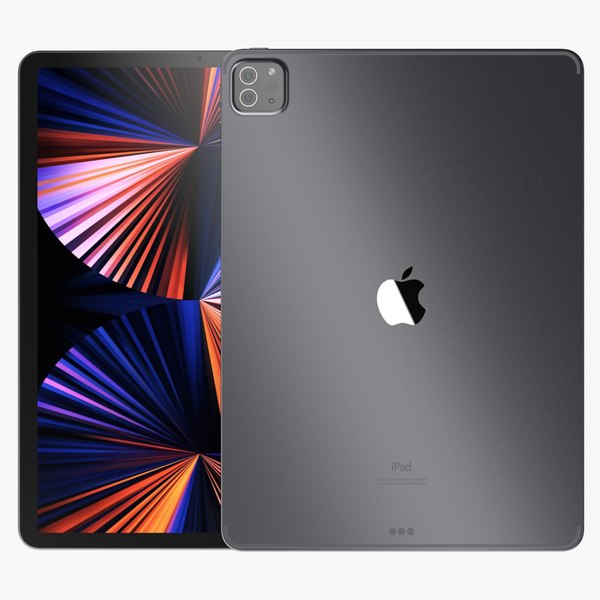
Po st w as generated by GSA Con te nt Gener ator DEMO!
These actions, and their concentrate on transparency, openness, fairness, and inclusion, have influenced my perspective on the problems surrounding today’s workshop, and I’m excited to be speaking here because it’s given me a chance to tie together some of my ideas on these ideas and their relationship to machine learning. We have a very interesting group of people right here at this time, with very various backgrounds, and I’m looking ahead to seeing what comes out of their interactions. Since the primary impetus for this workshop was President Obama’s ninety-day review of massive data, I need to start out by talking about a couple of definitional points surrounding large knowledge and its widespread makes use of. What is huge data? For something so spectacularly pervasive and trendy, I’ve heard this query surprisingly typically. Initially, I assumed this was as a result of no one knew (or not less than agreed upon) the answer. In this definition, volume refers to the amount of data in question, velocity refers back to the velocity with which that data can be obtained and/or processed, whereas variety refers to the vary of various information varieties and sources.
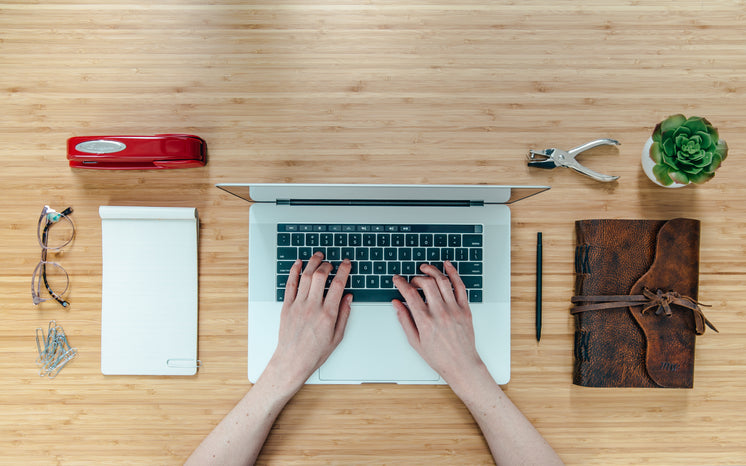
Nowadays, I think the explanation I hear this query so usually is that a few of probably the most salient properties of huge information, as it’s commonly construed, make individuals very uncomfortable. Yet, these imprecise, catch-all definitions don’t spotlight these disquieting properties. For instance, there’s little in these definitions - particularly Gartner’s - that distinguishes this “new” large information from the large information units arising in physics, for example. So why, then, are folks so worried about large data but not about particle physics? I think there are two reasons. In other words, not like the info sets arising in physics, the data units that usually fall under the large data umbrella are about individuals - their attributes, their preferences, their actions, and their interactions. That's to say, these are social data sets that doc people’s behaviors in their on a regular basis lives. The issue is not just dimension-we’ve at all times had big knowledge units - the problem is granularity. In different phrases, not only do these knowledge units doc social phenomena, they achieve this on the granularity of particular person people and their actions.

So why, then, does granular, social knowledge make individuals uncomfortable? Well, finally-and at the chance of stating the apparent-it’s because knowledge of this kind brings up issues relating to ethics, privateness, bias, fairness, and inclusion. In turn, these points make individuals uncomfortable because, not less than as the favored narrative goes, these are new points that fall outside the experience of these these aggregating and analyzing large knowledge. But the thing is, these points aren’t actually new. Sure, they may be new to pc scientists and software engineers, however they’re not new to social scientists. best computer science papers of many issues I really like most about working in computational social science is that, by definition, it’s an inherently interdisciplinary area, by which collaboration is essential to make ground-breaking progress: social scientists provide important context and insight regarding pertinent analysis questions, information sources, acquisition strategies, and interpretations, whereas statisticians and pc scientists contribute expertise in growing mathematical models and computational tools.